Risto Miikkulainen
The University of Texas at Austin and Cognizant AI Labs
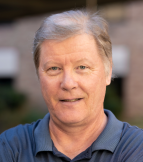
Generative AI of Decision-Making
AI has recently expanded from imitation to creativity, i.e. from replicating behavior that already exist to generating entirely new solutions. In addition to images and language, decision-making has emerged as a third such domain. The idea is to generate new behaviors, strategies, and designs that satisfy performance and cost objectives simultaneously. The approach is based on search, such as hill-climbing (i.e. reinforcement learning) and population-based search (i.e. evolutionary computation). Surrogate models are essential in making the search practical. I'll review a systematic such approach, evolutionary surrogate-assisted prescription (ESP), and present examples in pandemic response, climate change, and diabetes treatment. I'll also outline ongoing work on using large language models to generalize the approach to common-sense domains where traditional machine learning does not naturally apply.
Biography:
Risto Miikkulainen is a Professor of Computer Science at the University of Texas at Austin and Associate VP at Cognizant AI Labs. He received an M.S. in Engineering from Helsinki University of Technology (now Aalto University) in 1986, and a Ph.D. in Computer Science from UCLA in 1990. His current research focuses on methods and applications of neuroevolution, as well as neural network models of natural language processing and vision; he is an author of nearly 500 articles in these research areas. At Cognizant, and previously as CTO of Sentient Technologies, he is scaling up these approaches to real-world problems.
Risto is an AAAI, IEEE, and AAIA Fellow; his work on neuroevolution has recently been recognized with the IEEE CIS Evolutionary Computation Pioneer Award, the Gabor Award of the International Neural Network Society, and Outstanding Paper of the Decade Award of the International Society for Artificial Life.
Barbara Hammer
Bielefeld University
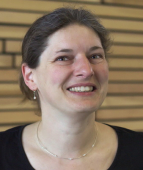
Explaining neural networks - deep and shallow
Variable importance determination refers to the challenge to identify the most relevant input dimensions or features for a given learning task and quantify their relevance, either with respect to a local decision or a global model. Feature relevance determination constitutes a foundation for feature selection, and it enables an intuitive insight into the rational of model decisions. Indeed, it constitutes one of the oldest and most prominent explanation technologies for machine learning models with relevance for both, deep and shallow networks. A huge number of measures have been proposed such as mutual information, permutation feature importance, deep lift, LIME, or Shapley values, to name just a few.
Within the talk, I will address two challenges of feature relevance determination:As models are increasingly used in everyday life, they face an open environment, possibly changing dynamics, and the necessity of model adaptation to account for changes of the underlying distribution. At present, feature relevance determination almost solely focusses on static scenarios and batch training. In the talk, I will target the question of how to efficiently and effectively accompany a model which learns incrementally by feature relevance determination methods(1,2). As a second challenge, features are often not mutually independent, and the relevance of groups rather than single features should be judged. While mathematical models such as Shapley values take feature correlations into account for individual additive feature relevance terms, it is unclear how to efficiently and effectively extend those to groups of features. In the talk, I will discuss novel methods for the efficient computation of feature interaction indices (3,4).
(1) F Fumagalli, M Muschalik, E Hüllermeier, B Hammer: Incremental Permutation Feature Importance (iPFI): Towards Online Explanations on Data Streams. Mach. Learn. 112(12): 4863-4903, 2023.
(2) M Muschalik, F Fumagalli, B Hammer, E Hüllermeier: iSAGE: An Incremental Version of SAGE for Online Explanation on Data Streams. ECML/PKDD (3) 2023: 428-445.
(3) F Fumagalli, M Muschalik, P Kolpaczki, E Hüllermeier, B Hammer: SHAP-IQ: Unified Approximation of Any-Order Shapley Interactions. NeurIPS 2023.
(4) M Muschalik, F Fumagalli, B Hammer, Hüllermeier: Beyond TreeSHAP: Efficient Computation of Any-Order Shapley Interactions for Tree Ensembles AAAI 2024.
Biography:
Barbara Hammer is a full Professor for Machine Learning at the CITEC Cluster at Bielefeld University, Germany. She received her Ph.D. in Computer Science in 1999 and her venia legendi (permission to teach) in 2003, both from the University of Osnabrueck, Germany, where she was head of an independent research group on the topic ‘Learning with Neural Methods on Structured Data’. In 2004, she accepted an offer for a professorship at Clausthal University of Technology, Germany, before moving to Bielefeld in 2010. Barbara’s research interests cover theory and algorithms in machine learning and neural networks and their application for technical systems and the life sciences, including explainability, learning with drift, nonlinear dimensionality reduction, recursive models, and learning with non-standard data.
Barbara has been chairing the IEEE CIS Technical Committee on Data Mining and Big Data Analytics, the IEEE CIS Technical Committee on Neural Networks, and the IEEE CIS Distinguished Lecturer Committee. She has been elected as member of the IEEE CIS Administrative Committee and the INNS Board. She has been an associate editor of the IEEE Computational Intelligence Magazine, the IEEE TNNLS, and IEEE TPAMI. Currently, she is involved in a number of large scale projects including the DFG collaborative research center on Construng Explainability, the EU Doctoral Metwork on Learning with Multiple Representations (LEMUR) and the ERC Synergy Grant Smart Water Futures.
Jakub Nalepa
Silesian University of Technology
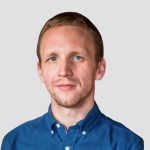
Unleashing Artificial Intelligence in Space: Exploring the What, Why, and How
Exciting advancements in remote sensing, AI, and edge computing are revolutionizing various scientific and industrial sectors by enabling in-orbit data processing. This breakthrough technology facilitates real-time applications like environmental monitoring, precision agriculture, disaster detection and in-orbit anomaly detection from telemetry data, among others. By integrating AI into space-based systems and edge devices, we can swiftly convert massive raw data, such as multispectral and hyperspectral images or multi-channel telemetry data, into actionable insights directly on board satellites. This not only accelerates data transmission to ground stations but also offers significant spatial scalability for Earth observation solutions and faster response to abnormal events detected on board a satellite. However, a multitude of challenges – related to the hardware constraints of in-orbit processing, to quantifying the capabilities of machine learning models without the real (target) data, or to limited/non-existing ground-truth datasets (as the world is not “labeled”) – persist, leaving the potential of machine learning algorithms untapped for various applications. In this talk, we will discuss the What, the Why and the How of bringing AI into space and will shed more light on the practical challenges and opportunities offered by such cutting-edge technology in the context of Earth observation and anomaly detection from satellite telemetry data, and will contextualize them within real satellite missions, such as OPS-SAT by European Space Agency and Intuition-1 by KP Labs. We will also delve into the current and future trends in satellite data analysis which are just round the corner. Ready to take off into space?
Biography:
Jakub Nalepa (Senior Member of IEEE) is an associate professor at the Silesian University of Technology, Gliwice, Poland, and he is the Head of Artificial Intelligence (AI) at KP Labs, Poland, where he shapes the scientific and industrial AI objectives of the company. He has been pivotal in designing the onboard deep learning capabilities of Intuition-1 and has contributed to other missions, including CHIME, Φ-Sat-2 and OPS-SAT. His research interests include (deep) machine learning, medical imaging, evolutionary machine learning, hyperspectral data analysis, signal processing, remote sensing, and tackling practical challenges that arise in Earth observation to deploy scalable solutions. He was the general chair of the HYPERVIEW Challenge at the 2022 IEEE International Conference on Image Processing, focusing on the estimation of soil parameters from hyperspectral images onboard Intuition-1 to maintain farm sustainability by improving agricultural practices.
Jakub has been awarded the Witold Lipski Prize (2017), the POLITYKA Science Award (2020), and has been shortlisted on the AI Innovation of the Year (Solutions Provider) list, The AIconics Awards (2021) (London, UK).